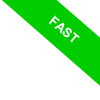
Difference Between Accuracy and Precision in Measurements
When measuring something and assessing error, it's crucial to understand that accuracy and precision represent two different aspects:
- Precision
Precision refers to how close repeated measurements are to each other around the average. Generally, the lower the relative error, the higher the precision of the measurement. Precision is mainly affected by random errors and can also be measured by the percentage error. However, it’s worth noting that precision alone doesn’t ensure accuracy, which is the closeness to the true value. A measurement can be highly precise yet still far from being accurate.A helpful analogy is a target. Imagine throwing darts: if all the darts cluster closely together but miss the bullseye, your measurement is precise (the throws are consistent) but not accurate (they're far from the true value).
- Accuracy
Accuracy shows how close the average of your measurements is to the actual or true value. A measurement is accurate when the average value closely matches the real value, indicating an absence of systematic errors. However, an accurate average doesn’t always mean the individual measurements are precise.To further illustrate, let’s revisit the target analogy. If your darts are scattered but mostly around the bullseye, you've achieved accuracy (they're near the true value) but lack precision (the throws aren’t consistent). Even if some darts land farther from the center, their average position is right on target.
Understanding the distinction between precision and accuracy is fundamental in any scientific field, as a measurement can be accurate, precise, both, or neither.
Returning to the target analogy, the ideal scenario is when all darts cluster closely around the bullseye. This represents both accuracy (near the true value) and precision (darts are close to each other).
The least desirable scenario is when the darts are widely scattered and also far from the center.
Grasping these concepts helps improve the quality of measurements and make more accurate assessments.
Practical Examples
Here are some practical examples to help clarify the concepts of accuracy and precision in measurements:
Example 1
Imagine you’re measuring the temperature of an oven set to 200°C using a thermometer.
You take five consecutive readings and get the following values: 198°C, 202°C, 201°C, 199°C, and 200°C.
The average of these measurements (200°C) is close to the actual oven temperature, indicating the measurement is accurate.
$$ \bar{x} = \frac{198 + 202 + 201 + 199 + 200}{5} = 200 \, °\text{C} $$
The readings are close to each other, with only a 2°C variation around the mean, indicating high precision.
The absolute error is
$$ e_x = \frac{202-198}{2} = 2 \, °\text{C} $$
The relative percentage error is
$$ \epsilon_x = \frac{e_x}{\bar{x}} \cdot 100 = \frac{2 \, °\text{C}}{200 \, °\text{C}} = 1.0 \% $$
In this case, the measurement is both accurate and precise, as the average is near the true value and the individual measurements are closely grouped.
Example 2
Now consider a different scenario. The oven temperature is still set to 200°C, measured with a thermometer.
You take five consecutive readings and get the following values: 195°C, 205°C, 198°C, 203°C, and 199°C.
The average of these measurements is 200°C, close to the actual temperature, showing good accuracy.
$$ \bar{x} = \frac{195 + 205 + 198 + 203 + 199}{5} = 200 \, °\text{C} $$
However, the individual values vary widely, with a 10°C difference between the lowest and highest readings, indicating low precision.
The absolute error is
$$ e_x = \frac{205-195}{2} = 5 \, °\text{C} $$
The relative percentage error is
$$ \epsilon_x = \frac{e_x}{\bar{x}} \cdot 100 = \frac{5 \, °\text{C}}{200 \, °\text{C}} = 2.5 \% $$
In this case, the measurement is accurate (the average is close to the true value) but not precise, as the readings are quite spread out.
Example 3
Let’s revisit the previous example. The oven is still set to 200°C.
You take five consecutive readings and get these values: 190°C, 191°C, 190°C, 192°C, and 191°C.
The readings are very close to each other, with only a 2°C range, indicating high precision.
However, the average measurement is 190.8°C, far from the true value of 200°C, making it inaccurate.
$$ \bar{x} = \frac{190 + 191 + 190 + 192 + 191}{5} = 190.8 \, °\text{C} $$
This suggests a systematic error, likely due to a calibration issue with the thermometer.
In this case, the measurement is precise (the readings are close together) but not accurate, as the average significantly differs from the actual oven temperature.
The absolute error is
$$ e_x = \frac{192-190}{2} = 1 \, °\text{C} $$
The relative percentage error is
$$ \epsilon_x = \frac{e_x}{\bar{x}} \cdot 100 = \frac{1 \, °\text{C}}{190.8 \, °\text{C}} = 0.5 \% $$
Example 4
Now imagine you’re measuring the temperature of an oven set to 200°C. The same oven...
You take five consecutive readings and get these values: 160°C, 200°C, 170°C, 195°C, and 165°C.
The average measurement is 178°C, which is far from the true value of 200°C, indicating low accuracy.
$$ \bar{x} = \frac{160 + 200 + 170 + 195 + 165}{5} = 178 \, °\text{C} $$
Moreover, the individual readings vary widely, with a 40°C range between the highest and lowest values, showing low precision.
The absolute error is
$$ e_x = \frac{200-160}{2} = 20 \, °\text{C} $$
The relative percentage error is
$$ \epsilon_x = \frac{e_x}{\bar{x}} \cdot 100 = \frac{20 \, °\text{C}}{178 \, °\text{C}} = 11.23 \% $$
In this case, the measurement is both inaccurate (the average is far from the true value) and imprecise (the readings are widely dispersed).
In conclusion, these examples highlight the importance of both accuracy and precision in measurements.
However, achieving high levels in both isn’t always necessary; it depends on your goals. Depending on the context, focusing on one or both may be appropriate, depending on the specific requirements of the measurement.